My Digital Gut: Making Nutrition and Healthcare Personalized, Predictive, and Preventive
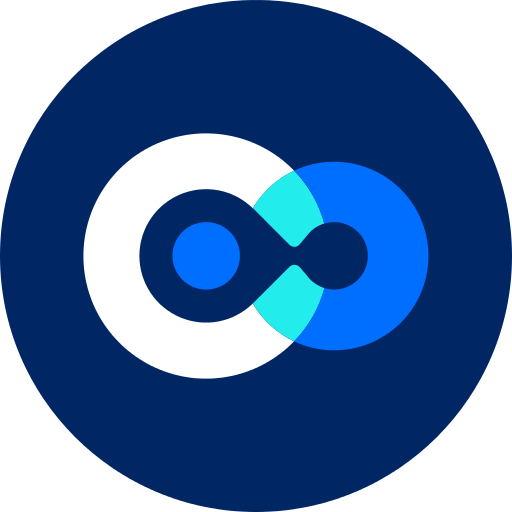
ISB Associate Professor Dr. Sean Gibbons delivered a Research Roundtable presentation and highlighted human gut microbiome research being conducted in his lab, including the development of a new tool called My Digital Gut. Watch his presentation by clicking play on the video above.
The human microbiome – the trillions of microbial cells that live on and in our bodies – is unique to everyone. Even identical twins have microbiome compositions that differ widely.
ISB Associate Professor Dr. Sean Gibbons has spent the past few years looking at how individuals respond differently to foods and exploring how we can leverage what we know about the microbiome to predict that variation.
In 2020, the Gibbons Lab released a state-of-the-art software platform called MICOM (a portmanteau of MIcroobial COMmunities), allowing researchers to build metabolic networks for a specific person.
MICOM models are initialized by data on an individual’s microbiome composition and dietary intake. Researchers can then take a person’s model (i.e., a digital twin of their gut microbiome) and simulate how adding probiotic organisms or changing dietary inputs (broccoli, bananas, prebiotic supplements, etc.) might impact the metabolic output of their microbiota.
Worldwide, many microbiome researchers have adopted MICOM as a research tool. MICOM has been downloaded more than 300,000 times, cited over 140 times in scientific publications, and enables researchers to run in silico experiments that would be nearly impossible to conduct in the lab.
The Gibbons Lab and collaborators have used MICOM in several projects that have yielded interesting and novel discoveries, including:
- Predicting if a pathogen or probiotic will “stick” in an individual’s gut,
- Finding that short-chain fatty acid (a molecular byproduct from microbiota consuming dietary fiber) production varies widely across people with the same diets, and
- Determining that individuals who produce higher levels of butyrate, a type of SCFA, are more likely to lower their blood pressure when adding a specific fermentable fiber supplement to their diet, and
- Applying a precision nutrition approach to a large population to determine how individuals will respond to different diets.
Today, Gibbons is building a new precision nutrition platform called My Digital Gut. My Digital Gut will be powered by MICOM and leverage the gut microbiome to make nutrition and healthcare personalized, predictive, and preventive. Once optimized and rigorously validated in clinical trials, Gibbons sees My Digital Gut as an essential tool that enables a new class of life-changing precision interventions.
Gibbons talked about all this and more during an ISB Research Roundtable presentation. You can watch his talk here, or by clicking play on the video above.
Learn how to become a My Digital Gut beta tester by emailing ISB Development Director Erika Kreger.
Video Transcript
Below is the video transcript of Sean Gibbons’ presentation and his Q&A conversation with Nitin Baliga.
Nitin Baliga:
Most of you have heard from news outlets that the human gut microbiome is an absolutely fascinating new dimension of human biology. It turns out there’s probably more cells that are microbial than our own in our bodies. So it really begs the question of what does it mean to be human? And this is a relatively new area of study and it’s of great interest to scientists because the microbiome has been associated with all kinds of health and disease. Sean is a leader in this field, and you are going to enjoy this presentation from Sean. So without taking any more time, it’s my pleasure to hand this over to Sean.
Sean Gibbons:
Thanks so much, Nitin, and I’m happy to be with you all today. Let me quickly share my screen.
Yeah. As Nitin kindly introduced, I study the microbiome. I’m interested in the bacteria that reside in and on your body and how they influence your phenotype, how you respond throughout your life to diet, to drugs, to all kinds of stuff. But today I’m going to talk a bit about our ongoing work in trying to understand how individual people respond differently to foods and then how can we leverage our knowledge of the microbiome to predict that variation. So as Nitin pointed out, we are perhaps more microbial than human. In fact, we’re about one-to-one about as many microbial cells in and on our bodies as there are human cells, about three to 4 trillion of each. Although I don’t want to overstate things because microbial cells are much, much smaller than human cells between 100 to 1,000 times smaller.
So if you could hold your microbiome in your hand, it would only weigh about half a pound. But by the numbers they’re about on par, depending on when your last bowel movement was, you might be more human than microbe. However, when it comes to the genetic capacity of the microbiota, they really truly do swamp us completely. The human genome has about 23,000 genes, and the microbiome contains something like 2 to 4 million unique genes, and many of those genes end up being important for our health and physiology. We’ve, over the course of our evolution, outsourced some of the functionality of our bodies, including the digestion of certain compounds in food to these microbes. And it’s part of what makes us all unique. We each have a completely unique microbiota, and that’s including identical twins, even identical twins who share the same genome, they have microbiomes that are almost as different as two strangers, maybe a little more similar than two strangers, but very distinct.
So this is a really unique and distinct part of our bodies that shapes how we respond to food. And we’ve known for a while now that this is a big effect. This is now almost 20 years ago, an experiment by Peter Turnbaugh in the Gordon lab at Wash U. They took poop from obese humans and from lean humans, and they did what was called a fecal transplant, which is that they took that stool from humans and they transplanted it into germ-free mice that had been reared in the absence of microbes, the bubble boys of mice. There was no microbes on them until they were exposed to this human feces, which colonized their guts. And then they fed these mice the exact same diet. What they found is that the mice that received a fecal transplant from the obese humans ended up gaining weight, more weight than the mice that got the lean microbiota.
They were eating the same exact thing. So the microbiomes of these mice, even though these mice were essentially clones of one another, they essentially share the same genome, was able to reshape how they responded to their diet. And this was a very broad result, right? It’s a hammer. You’re taking an entire community of microbes and you’re inserting it into an organism and looking for changes in their phenotype. But we didn’t exactly know what was driving essentially the weight gain in this case. And without that mechanistic level of understanding, it becomes difficult to translate this result into more targeted therapies or interventions. And so that’s a lot of what we focus on. And our group is trying to understand how to map the ecological composition of the microbiota to the functional outputs of the system that matter to us. And one of the main things that microbes do that matter to our bodies is they produce small molecules or metabolites.
Now, much of what they do for us is in that, essentially we eat a bunch of things that go into our mouths, dietary compounds, they travel through our system, we absorb what we can with our own genetic capacity, and then the rest the microbes get access to and they transform those molecules into thousands and thousands of bioactive compounds that then we can absorb into our own bloodstream and they can have distal effects throughout our body to all of our organs. And in fact, a couple of years ago, Christian Diener in the lab published a paper in Nature Metabolism, where we found that 44% of all the metabolites circulating in your bloodstream are related to ecological variation, person-to-person variation in the composition of your microbiome. So this was a much stronger effect than the genome, in fact, where about 10% of metabolites were associated with genetic variation.
So we know it’s a huge, huge driver of the small molecules circulating in our blood. But the challenge is because the microbiome is so complex and so vast and there’s so many genes, how do we build a translation to understand this conversation that’s happening between host tissues and the microbiota? We need a Rosetta Stone, right? This object that allowed us to translate hieroglyphics into Greek. We need something similar to understand this conversation. And in essence, that is essentially what we’ve been trying to build over the last few years. And to get into the details of exactly how we try to make this connection and understand this language, we have to talk a bit about enzymes, which are the workhorses of biology. Enzymes are essentially proteins and their function is to catalyze biochemical reactions. And to break that down, they essentially bind to metabolites.
So this green thing, for example, and when it binds, it can make some tiny conformational change that maybe splits up a molecule into two, or it combines two together to make one and spits those things out. So it transforms stuff, it takes inputs and has outputs. It creates small molecules from small molecules and reactions that might take thousands of years to occur on their own in a solution, an enzyme can accelerate the rate of that reaction to almost instantaneous speeds. So they are these almost magical biological entities that drive all of the chemistry of our bodies. And this is how we understand metabolisms, to understand these enzymes and the inputs and outputs to those enzymes. And in fact, over the past century, we’ve done a ton of work as a scientific community to understand this metabolism. And we’ve mapped out this vast map of metabolic transformations that are occurring in biological systems.
This is a picture of what we would call central metabolism, right? The central pathways that sustain life. And we really understand central metabolism quite well. That isn’t to say there isn’t more to discover. There’s actually an almost unfathomable amount of peripheral metabolisms on the edges of this network that we still haven’t worked out, but at least the things that are crucial to life, day-to-day survival, the central parts of metabolism, that’s pretty well understood for bacteria, for mammals, for other things. So how do we measure this functionality? What is the best unit to think about? Well, this little video here is showing us a metabolic network in a host cell, so a human cell for example. And it’s essentially a central metabolism of carbon taking in sugar, glucose and turning it into NADH, which is reducing power or electrons and energy ATP. And we could measure the individual enzymes that are responsible for these different metabolisms, so the genetic capacity of these enzymes, the genes that encode them.
We could sequence the genomes and see what enzymes are there, and that tells us something about metabolism. We could also measure proteins, which is the manifestation of those genes, the actual entity that’s doing the transformation. We can measure their concentrations inside of a cell. We can measure the metabolites themselves, their standing levels in a snapshot of time in a cell. But what I’m actually more interested in is something more dynamic. We actually want to know the flux of metabolites. What is moving through these networks? A concentration may not tell you that much about how important a metabolite is if it’s being produced at a really high rate, but also consumed at a really, really high rate. If you just took a snapshot measure of a given metabolite, it might not look like it’s that abundant, it might not look that important.
But it might be the central node in an entire network, and you’d only know that if you had measured the flux, how much of it is passing through the system? And that’s what this video is showing is the flow and flux through this biochemical network. And it turns out that we can build a map of this flux for an entire microbial community, at least for the central metabolism I was talking about before. What this hairball plot is showing is actually a mechanistic model of the metabolism of an entire person’s microbiome. Every one of these regions of high density is an individual organism, and you can see that there’s a lot of metabolic reactions happening inside of that cell. All these little lines are different metabolic reactions, there are a few reactions that pass between cells. So we can see that certain things are shared or cross-fed between organisms, and then there are other metabolisms that are at a community level that are external to the cells and that everyone contributes to the degradation of fibers or mucus and so on.
And this particular network contains almost 70,000 reactions, almost 50,000 metabolites and hundreds of thousands of edges or connections. And in a sense, this is our Rosetta Stone. It’s a very complicated Rosetta Stone, but it is a mechanistic model that we can build of a microbiome that we can play with and look at the inputs and the outputs of and make precision or even personalized predictions about how people respond given a dietary input. And so what is the engine here that is actually allowing us to build these big complicated hairball networks? Well, this is a software package called MICOM, which is a portmanteau of microbial and community, MICOM. And it was designed by Dr. Christian Diener, who was a research scientist in our group until recently. He now runs his own lab at the Medical University of Graz in Austria, but brilliant scientist who built this really complicated platform that allows us to construct these metabolic networks.
And what do you need for this platform? You need to have some information about the composition of someone’s microbiome. So you need to sequence their microbes and look at the abundances of each taxon that lives in that person’s system, that gives you a composition. You also need to input a diet so knowing something about what that person’s eating, if they’re an American, maybe you can assume they’re eating a standard American diet. Or if you have precision information like personalized dietary information, you can add that to the model as well. And I’ll skip the nitty-gritty details in this talk today, but you can read the paper if you’d like. At the end of the day, you get essentially all of the interactions between organisms, the cross-feeding, you get the consumption of metabolites from the diet, and you get the net production of microbial metabolites that are available to us or to the host to absorb into our bodies.
And this is a very well-used tool. The scientific community is currently using it as a research tool with hundreds of thousands of downloads and 130 citations. And these kinds of models we’re calling microbial community scale metabolic models or MCMMs for short. To give you a quick example of how we can use these models as a research tool, they enable us to do experiments that would be really hard to do in the laboratory. So for example, this is one person’s microbiome, right? And each one of these names is a different microbe, a different organism living in this person’s gut. And in the model, we can knock out each organism one at a time and then simulate the rest of the community and see what happens to the growth of all the other organisms in the system to try to look at competitive interactions or even cooperative interactions between things.
So in this particular person, if you knock out this organism, Bacteroides, it’s a genus of microbe, you find there’s a bunch of red edges. And what that is telling us is that the growth rates of many other organisms in the system increase if you remove Bacteroides. So it seems to have a competitive interaction with many, many other taxa in the system. However, other bugs like Akkermansia muciniphila, if you knock it out of the system, a lot of the growth rates of other bugs in the system go down. So it seems to be like a keystone species that helps other things grow. It makes sense because this particular bug likes to break down mucus in the mucus layer of our intestinal epithelium, which liberates a bunch of nutrients that other microbes can eat. So this is really fun. It’s a cool research tool. We can use it to explore the interiors of these complex interacting communities, but it’s hard to validate what’s going on, right?
Is the model true? Is it medically relevant? Can we actually validate this and look at outputs of the model that might be useful to us as humans rather than just as a research tool? So that is where I go next, I’m going to tell you a couple of vignettes about ways we’ve tried to validate our modeling platform in order to maybe find some use case that we might be able to deploy in the near future. So this is a story that’s driven from Alex Carr, a great PhD student in the group along with Christian who I mentioned before, and Nitin Baliga who you just saw, a collaborative work here. And what Alex is trying to ask in this particular project is, so before I told you, if you had a community of microbes in a person’s gut, and if you knock them out individually, what happens?
Well, what if you have an organism that isn’t living in that person’s system and you try to put it in? What happens then? Does that organism grow? Does it not? Does it bounce off or does it stick? This is a question that is really hard to answer, and it’s what our tool enables us to get a handle on. So here Alex is invading someone’s model with a pathogen, actually Clostridioides difficile, which causes C. diff infection, diarrhea, essentially. It’s a really common disease, especially in hospitals, but many of us carry C. diff benignly. It can live inside of our gut without causing problems and just waits around until we get sick or we take antibiotics and then it can cause disease. But we want to understand when and how and why does it colonize? So how do you test such a model? Well, you can do a lot of different things.
So one is to use in-vitro communities. So if you grow little microbial communities in the lab, you can then try to invade them with C. diff and then see what happens to C. diff’s growth. So this is now a collaboration with a researcher named Dr. Ophelia Venturelli. And Dr. Venturelli works in these in-vitro systems. And she had a bunch of communities, between 2 and 14 species of human gut microbes that she could grow in the anaerobic chamber in-vitro and invaded them with C. diff. And so we had her experimental results looking at C. diff’s measured growth rate in these different communities, and then we could simulate the same conditions in our model, and we find really striking resonance between the two. So just to give an example, on the X axis here is the number of species in one of these toy communities that she’s built.
Now the Y axis is the biomass after 48 hours of C. diff, which is its integrated growth rate over 48 hours. And what we see in the experiments is that there’s a steady decline in the ability of C. diff to grow as you increase the diversity of the ecosystem. That makes sense to us ecologically, because our commensal microbes can compete with these organisms for resources, for nutrients, and if they can suck up all the resources, it becomes more difficult for this other organism to grow. And that’s what we see in our model. We see the same exact thing, in more diverse communities it’s harder and harder for C. diff to grow. We can even compare the biomass production itself to a biomass production predicted from our model if we integrate over time and we see pretty good predictive power. We can explain about half of the variability in experimental biomass in these systems.
But what about in a living, breathing human being? This is an experiment we can’t explicitly do. You can’t give someone C. diff for good reason because it could make them very sick. But we can maybe use data from the wild that we can find where this happened naturally. So in this particular time series, this is a person who sequenced their microbiome over the course of a year. They had a food poisoning event here at this era, which is this red spike. They had a spike in Salmonella, which was a proteobacterium, this red colored organism. And at that same time point, they were colonized by Clostridioides difficile, and this was validated experimentally. Well, we can invade this person’s microbiome with C. diff throughout the entire time series in silico in the computer, and see what happens to its growth in our model. And when we compare the model to the measurement, we find that no matter what we do, we cannot get C. diff to grow in the time before this food poisoning event.
But afterwards we do see prediction of growth, and the predictions don’t perfectly match the measured amount of biomass, which is in blue. Blue is the measured abundance of C. diff and green as its predicted growth from the model, but it’s pretty good. We’re actually pretty happy with this result. So in this study as well, we wanted to ask, not only could we predict whether C. diff would invade a given person’s system, but could we rescue people? Could we actually find ways to protect them from C. diff invasion? And there just so happened to be a recent study from a company called Vedanta where they applied a cocktail of probiotics called VE303 to a patient population with recurrent C. diff infections. Recurrent C. diff is really tricky. You take antibiotics, but it keeps coming back again and again and again. And these infections can actually kill people.
And in the past we’ve used fecal transplants like I talked about in the mice to cure people of this infection. And that works great, but the FDA doesn’t like fecal transplants because it’s messy. You can’t reproducibly manufacture poop, so they want a cocktail. And this particular cocktail showed efficacy in a phase two trial, so it did do better than a placebo at treating recurrent C. diff. And we knew which organisms were in this cocktail. Unfortunately we missed two of them. So for six out of the eight bugs that were reported in this cocktail, we could find metabolic models that we could pull down to build an in silico probiotic intervention. So we could give this six member version of the cocktail to a population of people in the computer and see if it protected them from this in silico invasion with C. difficile.
And long story short, it did. So here on the Y axis is the relative growth of C. diff in the presence or absence of the probiotic. So it’s dividing the growth in the absence versus the presence. And so if you’re below the line here, it means that your growth is being suppressed by the probiotic. If you’re above the line, it means you’re being stimulated by the probiotic. And there’s only one case where we found C. diff being stimulated by the probiotic. Most of the time people showed suppression and that suppression was correlated on the X axis here with the average growth rate of the probiotic organisms in that person’s system. So the more the microbes were growing in that cocktail, in that person’s gut, the more they suppressed C. diff. And if you want to look at the mechanism of how this was actually working, we found that the closer this cocktail of bugs got to what C. diff was eating, the closer they got to its ecological niche within the context of that person’s system, the more they collapsed its growth rate to zero.
So if these organisms could compete for nutrients for metabolites successfully, they could essentially inhibit C. diff growth. We think this is really promising, and there’s a whole range of applications in terms of precision probiotics or disease risk prediction that could be enabled by this type of approach. The next vignette that I’ll quickly transition to is another use case for this modeling, going beyond predicting the growth of organisms in a system to predicting the metabolites that are produced by our microbiota. And there’s a particular set of metabolites that are super important that are made by our microbes that are called short-chain fatty acids. To a first approximation, you can think that the microbiome is a machine that ferments fibers into these short-chain fatty acids, and these organic acids are really important for our physiology and our health.
But the thing is, if you feed a bunch of people a banana which has inulin, which is one of these fibers, you get very different short-chain fatty acid profiles out the other side. And until now, this was an unsolved mystery, but I think now we have a way of predicting this inter-individual variability in these really important molecules. And this is work driven by Nick again, along with Kat and Crystal in the lab who are running our experiments, our poop soup experiments, which we’ll talk about. Christian of course, and then our collaborators at Fred Hutch, Johanna Lampe, and Lisa Levy for recruiting our stool donors for this study. So of these short-chain fatty acids, there’s one particular that I’ll call out and that is butyrate. Butyrate is the most important cardiometabolic drug that you’ve probably never heard of. That’s the title of the slide because I think it’s very true.
This is a molecule that has such vast impact on our physiology across all the systems of the body. And most people don’t know what it is, right? But it probably does more for you than many drugs that are on the market today. For example, it’s known to affect insulin resistance and glucose homeostasis. It can reverse pre-diabetes or prevent metabolic syndrome. It helps with weight loss, it helps with lowering inflammation, it reduces risk of cardiovascular disease and arthrosclerosis and stroke. So having more butyrate around is generally a really, really good thing for your health. But a lot of us in the western world who eat a low-fiber diet are actually really depleted in the production of this particular compound. So how do we understand the differences between people in the production of these short-chain fatty acids? It’s really hard to measure them in the wild in the gut because like I was talking about with fluxes, they’re produced at a really high rate by our microbes, but they’re also consumed at a very, very high rate by our intestinal cells.
They actually suck up butyrate in particular really fast. It’s their preferred nutrient source, even more so than blood glucose. So butyrate is rapidly consumed by our intestinal cells, converted into biomass and into CO2, and then it really doesn’t make it into the bloodstream that much. So trying to measure it in blood or stool, you almost can’t see much of it. It’s very noisy, it’s the flux that is really what we care about. So to measure this flux in the absence of host interference, we have to take poop outside of the body and make it into soup or what’s also called an ex vivo experiment where we mix poop with PBS buffer and then we add it to these deep 96-well plates, and we can then add in different macronutrient interventions like dietary fibers and then do time series metabolomics to empirically measure fluxes, measure the change in the abundance of metabolites over time.
And the slope of that line is the flux. It’s how much is being produced or consumed over time, and that’s what we really care about in terms of matching these things to our models. So what does the data look like? This is what it looks like right now. It’s not a perfect prediction, it’s still somewhat noisy, but the R value of the correlation coefficient is about 0.6 or translate that to the variance explained. It’s about 35 to 40% of the variance in inter-individual differences in production of butyrate or propionate, which is another short-chain fatty acid can be explained by our modeling, right? We can boot up these people’s guts in Silico, we can feed them the same fiber, make that prediction, that’s what the Y-axis is, and we can compare that prediction to our measured level of production for that person in-vitro, which is the X-axis.
So different people eat a banana, different stuff comes out, and it seems like we can quantitatively predict it to some extent. Now, an important thing to mention here is we’re not feeding some kind of statistical or machine learning model. We expect to actually do better from a prediction standpoint. This is a purely mechanistic model, and we’re not baking butyrate into the prediction at all, right? So we’re optimizing for biomass production in the community, and the butyrate production level is an emergent property of the model. So it’s amazing in some ways that we’re getting good prediction here. It means we really understand the metabolism going on in the system. So then how does this prediction relate to phenotypes in the host? We happen to have a large data set called the Arivale cohort, which many of you may be familiar with. It’s a bunch of adults from mostly the West Coast of the United States where we had many different measurements from their bodies.
We had their microbiomes, but we also had clinical chemistries from their blood, which are the kind of things you can go get taken at the doc, you can get your LDL cholesterol measured or blood glucose levels and so on. So we wanted to ask if we could predict short-chain fatty acid production across all of these people using their microbiomes and making a fairly simplifying assumption that they’re all eating, say, a standard American diet or European diet. How resonant are those predictions with these clinical chemistries? And what we find is that there’s a lot of resonance for butyrate, for predicted butyrate production, and that’s what the central bar in the heat map is. Don’t be intimidated by a heat map. The red means a positive relationship. The blue means a negative relationship. So as one thing goes up, another thing goes down. The rows are the different clinical chemistries.
These are the things you can get measured at the doctor. The first column is actually blood measurements of butyrate. So if you try to measure butyrate and blood, there’s no relationship between that and these clinical chemistries, the little stars on the middle column are telling you that this is significant association. So statistically it’s significant and the more stars, the more significant, essentially. The last column is propionate production, and we really don’t see much association between propionate in these labs. It’s really butyrate that seems to be driving things and we see what we expect. So it’s reported in the literature already that butyrate production is negatively associated with these bad markers of lipid metabolism or lipid health. So if you have more butyrate production, it looks like you have less LDL cholesterol, lower triglycerides, and so on. We also see relationships with insulin resistance, right? So if you have more butyrate production, you have a lower LP-IR score, which is an insulin resistance score and lower blood glucose levels.
So you have better control over your blood glucose when you have more butyrate production. And finally, inflammation. So it’s known that higher butyrate production reduces systemic levels of inflammation throughout the body. And indeed here in this cohort we see a negative association between butyrate production and blood CRP, which is a measure of inflammation. So this all matches our expectations with how butyrate should be shaping host physiology. Another validation that we’ve recently looked at is with a collaborator in Australia, Francine Marques, who is a cardiometabolic researcher and she’s interested in dietary interventions that can help prevent heart attacks and lower heart attack risk. And she had a recent study on looking at high amylose maize starch or HAMSAB with conjugated acetate and butyrate as an intervention against blood pressure to bring down blood pressure, essentially. The idea is these fibers are converted into these short-chain fatty acids, which have a dampening effect on blood pressure.
And what they found was a significant result. So when you compare placebo to this amylose treatment, you saw lower blood pressure in those people who were eating this resistant starch. But there were some non-responders, some people did not see reduced blood pressure, and in fact, they had a higher blood pressure after the intervention. So we wanted to see if we took their microbiome data and predicted butyrate production to this intervention in silico, could we see some resonance between the responders and non-responders? And Nick found a pretty striking difference. So essentially in those people who responded well to this intervention, they were producing between two and three times more butyrate for this intervention than the others. There was one person that was a bit of an outlier who we couldn’t explain, but by and large, we could see differences between the responders and non-responders. So we think this is really important for applying precision nutrition approaches to people.
So assuming all of this is working pretty well, which we’re pretty confident in, we’re pretty happy with a lot of this validation. What if we applied a precision nutrition approach to a large population? So this is a bit of a thought experiment. This is all in silico, we’re just experimenting in the computer, but we have this Arivale population. We can flip them between two different diets in the computer in the model, and then we can look at their responses to those diets. And if people aren’t responding as we want them to, like for example, bringing up butyrate production, we can try to add co-interventions alongside these diets to try to bring people back up to rescue, in effect the non-responders. And to give you the overview of the results, if you take people and feed them an average European diet, and here I think it’s the average Austrian diet, so it’s a northern European meat-heavy diet, and you flip them to a vegan high-fiber diet, you do see higher levels of butyrate production on the vegan diet as we would expect.
But there were subsets of this population that were non-responders. If you flip them to this vegan diet, they showed no difference in butyrate production. There were even regressors people who had a slight reduction in butyrate production when flipped to this diet. Now the blue is showing the optimal co-intervention. So it’s showing us how much we could rescue these groups if we added a simple co-intervention. And now the heat map, again showing a heat map, is telling us something about which intervention did best for which person. The non-responders are in green, the columns in green, these regressors I talked about are the columns in yellow, and now every row here is a different intervention. So it’s a different diet or a diet plus prebiotic fiber, which is B. pectin or inulin or probiotic intervention. So for the probiotic, we actually added up an organism called Faecalibacterium prausnitzii, and it’s a butyrate-producing bug that lives in your gut.
So what you see from just an overview of this, each column being a person, the black box is outlining the intervention that was best for that individual person, and basically there was no single intervention that was best for everyone. For some people they could be rescued by just adding a simple dietary fiber to their diet. So taking an inulin supplement for example, could really turn up butyrate in this particular person. For other people, it didn’t matter how much fiber you fed into their system, if they lacked the genetic capacity in their microbiome to produce butyrate, they weren’t going to make it. And so for them, they really required a probiotic intervention and the model was able to identify those people and perhaps allow us to deploy those interventions in a precision way. So this is some of the validation we’ve done so far.
How do we make these models better? We need better databases, and that’s coming. The scientific community is working on this. We’re trying to help as well, but that’s all happening and these models should only improve as our databases improve. We need to do a better job of integrating host metabolism, so building in metabolic models of host side metabolism from the epithelial cells or the liver and so on. This is all something we’re working on as well. We need better methods for optimization. You don’t need to worry about that, but we are, and again, as the models improve, so should the predictions. Finally though, as I end off on the scientific portion of this, better constraints on dietary intake are really important. And I’ll touch on a method that we’ve recently put out as a pre-print that we’re trying to use as a way to infer precision diet directly from data.
Diet inference is actually really tough. If you ask people to report their own diets, they’re often liars sadly. We’re much more likely to report eating a salad than we are eating a candy bar, so it’s hard to get really accurate information on diet. So Christian Diener in the group, he just developed a method we’re calling MEDI to directly extract DNA from stool that comes from the foods we eat. We eat things with DNA like plants and animals and fungi and some of that DNA survives passage through the gut. We also want to be able to map those organisms to their metabolic composition so we know how much fat and carbohydrate and so on is in these different organisms. This tree is showing a big evolutionary tree of all the different foods that we could find that have been sequenced, that are present in the human diet, hundreds and hundreds of organisms, we see that, for example, nutrient levels of the plants which are on the right here are enriched in carbohydrates.
The animals are more purple down here and they’re enriched in protein as you would expect. And then the fungi over here are enriched in carbohydrates. We’ve done a lot of tricks to make this work really well, and I think I’m running a little short on time, so I’ll skip some of the nitty-gritty details, which you can read are preprint if you want. We find that sometimes we get mismapping of organisms if we have really related types of foods. So species in the same genus sometimes get confused for each other, but we actually don’t think that’s a huge problem for dietary inference because the nutrient composition of biomass is actually pretty similar for two species in the same genus. It’s only when things become really evolutionarily distant from each other that you start to see bigger divergences in the nutrient composition. If we test our method against self-reported data, we’re actually getting pretty reasonable results.
So this is data from the Human Microbiome Project cohort, and the X-axis is over here on the right. It’s the frequency of intake of these various foods to the farthest right is the most frequent intake and to the farthest left is the least frequent intake. The Y-axis on these plots is the inferred amount of that food component from our model. So it’s the MEDI-estimated level, and we see that for fruits for example, we get pretty good prediction, right? If you report eating fruits every day, we see more fruit in your poop. And if you don’t report eating that much fruit, we don’t see that much in your poop. Same for red meat, same for vegetables. Some foods we’re really bad at still like shellfish, but part of that is probably because we don’t eat a lot of biomass when it comes to eating shellfish and shellfish might be really fragile, so it maybe breaks down more easily.
And so ultimately, yeah, we want to be able to someday use this as a way to port personalized dietary information directly into our modeling, and that should only make the models more accurate. So as I end off here, I will just quickly walk you through some vision we have for how do we take this beyond a simple research tool and turn it into something that people could maybe use one day? We’re still a ways away from this, but it’s in the process of becoming, and so I’ll share the vision. Basically, let’s just tell the story of two people, Jane and Jon Doe, and let’s say Jane and Jon both threw down some money for an expensive probiotic, let’s say Akkermansia muciniphila, which is one of these microbiomes you can currently buy on the market. And maybe Jon felt great, actually his digestion was improved.
He had really good response to this particular intervention, and Jane was meh. She didn’t see much difference. And then they hear about this idea of maybe booting up a digital twin of their microbiome or My Digital Gut, if you will, that leverages some of the technology I just walked you through, to try to ask why were they having these differential responses. So the idea would be to build some platform that people could log into and learn about their microbiome, about its metabolic capacity and manipulate it. Of course, you create some account, have some consent to research. We would hope this to be a citizen science thing where people’s participation would also help on the science side with understanding variation in response to diet better. To give you an idea of what we’re envisioning for this patient journey or this participant journey, Jane would get a poop sampling kit mailed to her house.
She would then send that off. Her data would be generated, we would sequence her poop. That data would then be integrated into our model. We would build a personalized representation of the metabolism of her microbiome in silico, and then we could add different interventions to her model. We could feed her different diets, different probiotics, different prebiotics, and then we could compare those outputs to a population of people. So how does Jane compare to 1,000 other people in the population? And then provide that information back to Jane. Potentially too, in a citizen science way, we might also think about collecting blood because if people were to intervene on their health through diet, eating different foods and they wanted to track changes in pre-diabetic markers or cardiovascular risk markers, and you want to see that the trend is going in the right direction, we could try to track that as well through measuring the blood. To give an idea of what this might look like, Jon and Jane are looking at their personalized output.
Maybe we want to flip them between a few different diets. So here are five different diets we can feed both of these folks and they showed variable responses. And here we’re just focusing on butyrate, for example, because we know we can predict it fairly well, but the population distribution is just some population of people that we can simulate a priori and we can embed Jane and Jon’s results on top of it. So the smiley faces is Jon’s result on top of this population. It looks like for Jon, the vegan high-fiber diet gives him the best butyrate production. He does a little better than the average person in the population on that diet, whereas the keto diet, he does actually worse than average on that diet. For Jane, it’s different. She actually does best on a Mediterranean-style diet, and she’s actually worse than the average person on a vegan high-fiber diet and would’ve only be through such type of simulation that you could easily do this type of choosing without trial and error in real life of switching between these diets.
So let’s say you had a diet that you thought was optimized in the model and you wanted to pick a co-intervention, like a prebiotic or a probiotic to put on top of that diet. Well, for Jon, let’s say he chooses the vegan high-fiber diet as his best diet and then simulates a bunch of possible probiotics for him, Akkermansia muciniphila gives him the biggest boost in the butyrate production. Well, as I mentioned earlier, that was the exact same bug that both Jane and Jon had purchased, and he actually felt good taking that probiotic. Jane, for example, she actually doesn’t do that great. She actually shows less butyrate production on her optimal diet when taking Akkermansia but Faecalibacterium gives her a big boost. So for her, that would be a more appropriate intervention. And ultimately, given that we validate these things in trials and so on, it would be great for Jane and Jon to be able to shop for their microbes in addition for themselves.
Hippocrates said long ago, “Food is medicine.” It’s just been difficult to do the translation to really make food intake a quantitative way to manipulate your health. As we move forward, we’re trying to build this My Digital Gut. The first thing we want to do is run trials. We want to run clinical trials that prove the precision microbiome-informed nutritional interventions give you a better result, say, in terms of blood pressure or any other thing versus some placebo or some randomized intervention. Eventually, we want this to be integrated into healthcare decisions, so a clinician maybe can guide people’s behaviors using this type of modeling approach. And if it all validates in the end, we will eventually hope to see people be able to use this themselves without having to go through any intermediary. But that’s perhaps the farther away future. With that, that’s the vision. That’s the science thanks to Alex, Nick, Kat and Crystal, Christian for all their hard work on this. Nitin Johanna, Lisa and Ophelia, the collaborators. And thanks to all of you for listening, and I will end there.
Nitin Baliga:
That was fascinating, Sean, as always. There’s a whole bunch of questions. I don’t think we’ll be able to get through all of them. So let’s get some basic definitions. I think a few people have asked this, how do you define a prebiotic and a probiotic? And someone said that there’s some rumors about postbiotics. So what are these terms?
Sean Gibbons:
Yeah, there’s a lot of words out there. So prebiotic, that is the food that microbes eat. So say a dietary fiber, which we can’t digest, but microbes can. That would be a prebiotic. Technically the definition is it’s an indigestible compound that promotes the growth of a beneficial microbe. That’s the definition of a prebiotic. A probiotic, that’s the microbe itself. So you’re giving the bug itself as an intervention. Postbiotic is the metabolites that are made by the microbe. So butyrate is an example of a postbiotic. You could give someone butyrate as a direct intervention, and there are people working on these types of things. Finally, there’s one more. It’s called a synbiotic, and that’s where you combine a prebiotic and a probiotic, so you have both the microbe and the food that it eats… I don’t know why I’m going off on fireworks right now.
Nitin Baliga:
Making fascinating comments.
Sean Gibbons:
Yeah, exactly. So those are the words, yeah.
Nitin Baliga:
And based on what you’ve described and papers I’ve read, people have different microbiomes based on what they eat and where they live and their medical histories and all of those things. So when you talk about these pre, post, probiotics or synbiotics, I would imagine even those would need to be tailored to an individual based on their resident microbiome. Is that correct?
Sean Gibbons:
Totally. Yeah, we see that in our simulations already. Like inulin works great in some people, but not so great in others, and that’s even just among Americans. But if you go to other parts of the world, yeah, the composition and the metabolic capacity of the microbiota varies substantially, whether you’re in India or China or Europe. So you really do need, I think, tailoring here to make it work well. And another quick aside is culture matters here too, you might tell someone in Louisiana that artichokes would be good for them, but maybe they’ve never seen an artichoke or they’ve never prepared one before. So we also need to design interventions that are culturally appropriate that use foods and compounds that would be familiar to someone that would actually be using them.
Nitin Baliga:
Very interesting. And in terms of administering these probiotics, some people are interested in knowing, is there a special delivery that is required. Does the probiotic get destroyed in the process of traversing to your gut?
Sean Gibbons:
That’s a great question. Some companies think more about this than others. There are different ways of preparing probiotics. You can freeze dry them, you can do other things to them. You can turn them into… Some are spore producers, so you can coax them to make spores, which are these tough seed-like structures that survive the stomach and lots of other things. So depending on how you prepare the probiotic, it can more readily passage through the upper GI tract, which is very harsh. There’s a lot of acid, there’s oxygen, so it can kill a lot of gut microbes. Alternatively, you can design capsules that only dissolve in the colon. So some of these capsules are treated with coatings that allow them to survive through the acidic stomach and small intestine and only open up when they arrive in the colon.
Nitin Baliga:
Interesting. Now, some diseases like colon cancer are known to have some risk association with the microbiome. And so in those testings that are currently done for colon cancer, is microbiome sequencing considered at all, do you know?
Sean Gibbons:
Not that I know of in the clinical tests. So the clinical tests, the sequencing tests for screening colon cancer, that’s more host DNA. So they’re sequencing for potential tumor-derived DNA that’s being excreted out into the colon. However, there are certain organisms that are enriched in colorectal cancer. The signature we often see is that colorectal cancer, you start to see organisms that tend to be associated with your mouth microbiome, like Fusobacterium that you almost never see in the colon, but you do see them in the case of colorectal cancer. And what I think is going on is these structures, these tumors that grow out above the mucus layer provide a landing zone for these biofilm-forming mouth-associated taxa to land on and grow. And many of those organisms cause inflammation and problems that could further potentiate cancer. So there is a lot of work in the area of the microbiome and colorectal cancer, but there’s no currently available clinical diagnostic that leverages the microbiome.
Nitin Baliga:
And going the other way in terms of other organs in the body and other locations where microbiomes play an equally important role, is there any effort to look at, for example, the oral microbiome or the skin microbiome and do similar things you’re doing with, seems like the gut microbiome is one of the harder ones you’ve selected, but great results of course.
Sean Gibbons:
Great question. Yeah, the microbes are everywhere. You can think of the human body as a donut, topologically, right? It’s one surface all the way down and out through your anus and to your skin. It’s all one continuous surface that’s the outside, and all of that surface is covered in microbes. 99% of all the bacterial biomass on your body is in the colon. So by mass, the colon is really where most of the action is. But of course, microbes and other parts of the body are important. Actually, Nick Quinn-Bohmann just had a paper out on the upper respiratory tract microbiome.
We did a meta-analysis in health and disease and showed that certain organisms are consistently enriched in disease or in health. There’s really cool work on the skin. Recently, a researcher at Stanford, Michael Fischbach had a paper where he showed that a skin microbe could be sensed by the immune system, and they were able to actually engineer cancer antigens, neoantigens of cancer onto that microbe, paint it onto the skin of mice, and this mouse immune system destroyed or eradicated the tumor. So it was like a tumor vaccine that was applied via just a paintbrush of microbes on the surface. So there’s lots of interactions going on. We mostly focus on the gut just because.
Nitin Baliga:
Yeah. Yeah. Super cool. So coming to My Digital Gut, someone is interested in knowing if you have changed your diet based on your microbiome analysis.
Sean Gibbons:
Yes. I eat more whole foods. I don’t eat processed foods as much as possible. I prepare a lot of my own meals and I try to bias my intake more towards plants. I still eat meat. I’m an omnivore, but I try to, for the most part, maybe five, six days a week, mostly eat just plants. So fiber and the diversity of plants you eat, the more, the better, and your microbes like that. And if you eat more of that, you get more short-chain fatty acids, the benefits weigh down to you. I’ve also started taking probiotics recently.
There’s some that are out there that I like a lot. I’ll call out a company, Pendulum, that I like a lot, and they have a few like Akkermansia and Clostridium butyricum that I do believe are actually effective probiotics. Most of the probiotic market is snake-oily. A lot of the organisms that are currently probiotics are essentially grandfathered in because they’re present in cheese or sauerkraut. But the next generation probiotics that are the actual bugs abundant in adult gut, there are now companies finally that are selling these as probiotic interventions. So I think that’s really exciting as well.
Nitin Baliga:
Very cool. I remember a paper from Eric Ham some time ago. I think you showed some results from that paper where the microbiome stayed relatively constant throughout, and the only time it changed was when they had that severe diarrhea, both him and his graduate student. And so when you take these probiotics, how likely is it that they become a part of your microbiome? I know your models can predict that, right?
Sean Gibbons:
Yes. So we can try to predict it with our models. Empirically, there’s not that many studies on this. Generally speaking, oftentimes when you take a probiotic, it just bounces off. As long as you’re taking it, you can detect it in your system, but if you stop taking it, it goes away. But there was a paper a few years ago in Cell Host & Microbe where they gave a bunch of people a bifidobacterium, a probiotic, and about one third of them, when they stopped taking it, it stuck around the other two thirds, it went away. They were able to predict it by finding that those people where it stuck, they actually lacked that particular organism in their gut to begin with. And the people who were it bounced off, they already had organisms that inhabited the metabolic niche of the probiotic. So it is the matching of metabolic niches and the modeling allows you a way to make those predictions.
Nitin Baliga:
Right. Unfortunately, we’re at the top of the hour. We’ll go a little over, but I do want to ask you one important question. There’s many questions. I encourage you to go back and read those questions. So one of the questions is, can anyone participate in My Digital Gut? Is there a cost involved? Are there any eligibility requirements, et cetera?
Sean Gibbons:
Yeah. So this is just a vision at the moment. We’re trying to build it. It’s in early days, but we are accepting philanthropic donations to support it. And in fact, we have enough right now where we’ve begun to build the platform. So if you’re interested in supporting this, My Digital Gut, and maybe becoming a beta tester, you could contact our Development team or go to my website and contact me and we can get you set up to help support that. But we’re in the early phases of building it, and we already have some very generous support, but we could use more, for sure.
Nitin Baliga:
Well, Sean, we could go on and on, but we have to end there. And thank you so much. This was absolutely fascinating, an eye-opener, and I think that clearly there’s the future of human health that’s being opened up by microbiome studies and thanks for leading the way. And thank you everyone for joining us today. I really hope you enjoyed Sean’s talk. I know, I’m sorry we couldn’t answer all of your questions, but do reach out to Sean and he loves talking to people and sharing his research and then answering questions. And as you mentioned, there’s a great opportunity to help fund My Digital Gut project that is now going from vision to execution. And those of you who would like to participate, you can find more information, as Sean suggested. And if you’d like to make a gift of $2,000 or more, you will receive an early invitation to sign up to be a My Digital Gut beta tester.
So if you would like to learn more or reply to any of the emails you received about the event, and one of my colleagues from the Development department will get back to you and keep an eye on your inboxes, there’ll be more research round tables like this one. As I said, it’s a quarterly series and there’s many other ISB events as well that you may find interesting and would like to participate in. And with that, I close today’s session. And again, thank you all and thank you Sean very much, and have a great rest of your day.